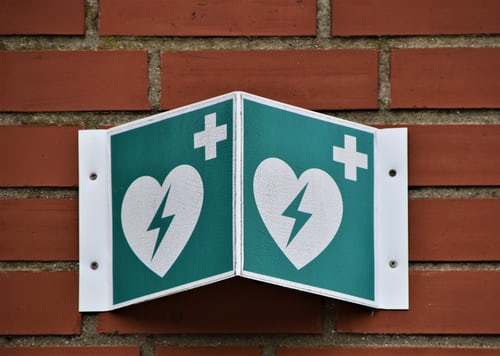
Machine Learning for Early STEMI Detection
I had the pleasure of speaking with Dr. Yiadom about her talk, AI is Here & is Your Friend: What Machine Learning Can Do For You in your ED, at this past year’s ACEP. Dr. Yiadom has a background in healthcare policy and Masters in public health from Harvard where she also completed residency at the affiliated program, Mass General and Brigham and Women’s Hospitals’. She completed a Masters in Science in Clinical Investigation at Vanderbilt University. Since joining the faculty at Stanford she has been developing a Machine Learning model that will allow a computer to predict which patients are candidates for an EKG before being seen by a human provider. The hopes that patients with a possible STEMI will be detected earlier and decrease miss rates.
Dr. Gowda: From what I'm understanding: There are patients who are not getting an EKG done within 10min arrival to the ER and some of these patients are STEMIs that could be caught earlier if they had an early EKG
Dr. Yiadom: Yes
Dr. Gowda: You developed an AI algorithm based on signs and symptoms such as CP, and other associated SS and age at Stanford that predicts when a patient needs an EKG
Dr. Yiadom: We are developing it using machine learning and statistical inference approaches.
Dr. Gowda: How did you become interested in this work? with diagnostic algorithms? chest pain? with AI?
Dr. Yiadom: I have always been interested in clinical operations. After residency, I did a lot of moonlighting and the variation in what prompted triage EKGs for physician interpretation across EDs started to worry me. So I started to look at this from an administrative policy perspective...then from a clinical process view...then I wanted to see the data on what was similar across EDs. After doing some research locally, I started to look at other EDs. Once we realized it was a broad problem in EM, I started to think about solutions. This was shortly after EHRs became the norm. I started seeing if we could use the computing power of the EHR to help. After a few courses and projects with informaticists, I ended up developing expertise in this area of chest pain evaluation, diagnostic algorithms, AI, and actively harnessing the EHR to improve care delivery reliability.
Dr. Gowda: How did you develop the AI algorithm? Does it use logistic regression? random forest? Did you work with anyone outside of the ER to develop it?
Dr. Yiadom: All of the above. We use lasso regression with random forest walking and cart to identify the most influential variables. Then we use logistic regression to quantify their influence. Those with statistical significance are included and we use the strength of the association (with operational process consideration) to rand the variable order in the model.
Dr. Gowda: Have you implemented the AI algorithm and studied the outcomes?
Dr. Yiadom: No. We are refining the model and doing work to understand how our influential variables are associated with ACS. We are starting work to translate and pilot the application of the model as clinical decision support.
Dr. Gowda: What are the challenges you found when developing the algorithm? exs: volume of data, missing data? Implementing the algorithm?
Dr. Yiadom: We’ve had challenges in all of these areas, however, the biggest is that is required multicentered data to 1) Have a large enough sample of patients to study a low-frequency event like STEMI, 2) Understand the influence of operational process and practice variation.
Dr. Gowda: It sounds like you chose Random Forest partially because providers would understand the significance of the variables chosen in the predictive model. Do you think that transparency will be important for implementing ML models?
Dr. Yiadom: Transparency is generally less important for ML models except for when you want to learn from them. Then using an approach that can share the most influential features can be helpful.
Dr. Gowda: What do you think some of the next steps are for this project? To your knowledge is there anything like this being used in ERs?
Dr. Yiadom: There are predictive models embedded in EHRs. There are CDS tools used in clinical practice, but we are not aware of a predictive model developed to mimic physician decision-making intended to advise a clinical decision for a timely sensitive care condition where this is only 10 minutes for a non-clinical provider to initiate a test. This is an “emergency care” specific niche where we find our work is unique.
Dr. Gowda: What is it like presenting via Zoom at ACEP? Was there as much discussion?
Dr. Yiadom: The talk was pre-recorded, but a few people reached out via email with follow-up questions.
Maame Yaa A. B. Yiadom, MD, MPH, MSCI
Associate Professor, Emergency Medicine
Stanford University