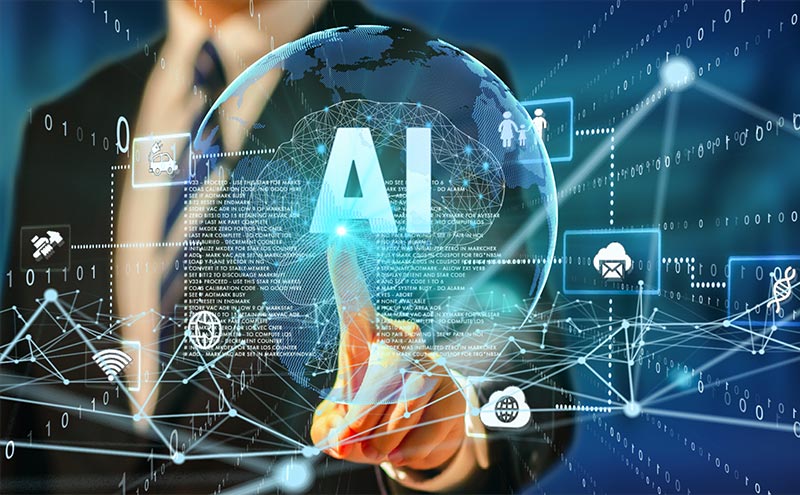
Exploring and Leveraging Artificial Intelligence
Chanel E. Fischetti, MD
Overwhelmed by the terms artificial intelligence (AI), machine learning, deep learning? Well, you’re in luck. I’m here to tell you, you don’t have to jump into being a know-it-all or expert anytime soon. Just familiarizing yourself with the terminology and functionality of what these terms can mean can help you orient to the currently evolving space of innovation and technology in medicine and science.
In fact, we are all active participants in AI technology daily: the inputs we give into our social media - the likes we give, the articles we read in the news - help train the models applied to us to keep suggesting things we will be interested in and avoiding things we may not be. In emergency medicine, AI has more important implications for highlighting vitals of a patient about to crash, bumping that head CT of the unexpected subarachnoid hemorrhage patient up the list of radiology reads, or even something as simple as language prediction in emails.
Broadly speaking, AI is an umbrella term that encompasses both deep learning and machine learning. The best example of machine learning is the RECAPTCHA you participate in anytime you go to purchase something. The inputs you’re making towards identifying the cars, bikes, and trucks are all contributing to machine learning: literally teaching the machine what to recognize as a bike or a truck. Deep learning takes it a step further. It requires much more data and uses prediction models to perform and produce an output or outcome. The best exam of this is prediction modeling Google has created for earlier detection of diabetic retinopathy. Scanning eyes to detect nuances not even recognized by the human eye.
But let’s say you have a grasp of the concepts but are curious: how do I get more involved or leverage this technology to my benefit? What are the applications in which I can think of using AI for my work or to my advantage.
Within the realm of ultrasound areas of ongoing AI research include, but are certainly not limited to: predictive modeling for B line detection on lung ultrasound, estimating cardiac ejection fraction from a cardiac ultrasound, or nerve bundle identification for nerve blocks. The power of AI, specifically within ultrasound, has important implications for our field as technology has outpaced the ability to keep up with the volumes of data being produced. Portable ultrasound machines are now more ubiquitous and affordable, yet one of the rate limiting steps in more widespread point-of-care ultrasound (POCUS) adoption is that often new users still don’t feel confident or comfortable interpreting their own images. AI will likely be the most immediate solution to bridge the gap between the innovation of technology and the current volumes of data needing quality assurance and review. The AI modeling and research that is currently being developed will be pivotal to help facilitate current educational and clinical gaps in diagnostic abilities and knowledge. Therefore, the next great push we can make in ultrasound education is in contributing to ultrasound AI models.
Feeling convinced yet? But still don’t know where to start? Well if you’re hoping to better understand the landscape, a quick Google search for AI or Machine learning 101 will generate many options for free and paid curricula online. The next step is to find a data scientist or computer scientist with whom you can collaborate or partner with on research. You don’t have to be an AI expert to get involved. Some of the best learning comes from collaboration and learning from others. If you’re connected to an academic medical center, the easiest way to do this is by connecting with any of these adjacent departments. Not ready for even a collaboration or partnership? AI models are only as good as the models they’re trained on. Therefore, another important part of creating good AI models is annotating and making sure that the datasets the models are training on are accurate. Consulting for an ultrasound company to annotate images is another meaningful way to get involved.
Wherever you decide to start, I think it’s important to understand that AI isn’t going away and will continue to be more integrated into our ultrasound machines, workflows, and diagnostics. So there’s no better time to jump right in and start learning and participating!